Episode #3: The Critical Role of Data Freshness in Business Decision-Making
Here are 5 key takeaways from the podcast:
Data Freshness Drives Quick Decision-Making: Fresh data helps businesses respond in real-time to changing circumstances, like adjusting promotions or stock levels during a flash sale.
Different Levels of Freshness: Data freshness can vary based on business needs: real-time, near-real-time, and batch updates—all essential for different types of decisions.
Real-World Applications of Fresh Data: Key industries like marketing, customer support, inventory management, and fraud detection rely on fresh data to stay agile and proactive.
Key Metrics for Data Freshness: Businesses should track latency, availability, completeness, accuracy, consistency, timeliness, and update frequency to ensure their data is fresh and reliable.
Overcoming Data Freshness Challenges: Best practices include consolidating data, automating collection and transformations, scheduling updates, and building scalable infrastructure to keep data fresh without sacrificing performance.
Dive deeper with this read
The Critical Role of Data Freshness in Business Decision-Making in 2024
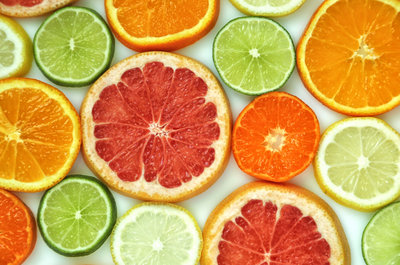
Podcast listing
Vadym:
Welcome back to The Data Crunch Podcast! I’m Vadym, Growth Marketing Manager here at OWOX, and today, we’re diving into a topic that’s essential for making good decisions fast: data freshness.
It’s all about how quickly data goes from being collected to being ready to use. And with me here is our Head of Marketing, Ievgen, to help us break it all down. How are you today, Ievgen?
Ievgen:
Hey Vadym, I’m doing great! As always.
Yeah, data freshness is one of those key pieces that makes all the difference between being responsive and playing catch-up, but also it’s about costs.
So I’m really excited to talk about it today.
Vadym:
Exactly, I think a lot of companies are used to just having data, but today it’s about making that data usable in real-time, or at least close to it. So, Ievgen, maybe start us off with the basics—why is data freshness so important in business decision-making?
Ievgen:
Well, it’s pretty simple. The fresher the data, the more it reflects what’s happening right now.
For example, let’s say you’re in e-commerce and running a flash sale. If you can see which products are selling quickly, you can adjust your promotions or stock in real time.
Without fresh data, you might miss those quick reactions and lose out on sales.
But again, there is the other side of things. We can refresh all reports every minute, but that would cost us a lot…
Vadym:
So, as I understand it’s all about being able to respond in the moment.
Now, what about different levels of freshness?
Not all the data can be real-time, Not all reports has to be real-time, right?
Ievgen:
Exactly. Data freshness can mean different things depending on your needs. There’s:
One it’s Real-time, where data updates instantly—like in stock trading.
Second it’s Near-real-time, which updates every few minutes or hours, and it’s useful for customer interactions or during promo campaigns like the upcoming Black Friday.
And finally, the data can be updated in Batches. This is where data is updated once a day or even once a week, which is often enough for strategic or operational planning, or reporting to C-levels, board meetings, etc.
So data freshness is about picking the level that fits your specific decision needs.
Vadym:
That makes sense. Now, I know you mentioned e-commerce, but are there other key applications where real-time data is really valuable?
Ievgen:
Definitely. Fresh data is crucial in a lot of areas:
Marketing Campaigns: If marketers can see data in real-time, they can adjust campaigns while they’re running.
Customer Support: With up-to-date data, support teams can see recent interactions and take actions faster.
Inventory Management: Fresh data helps companies track stock levels in real time, avoiding overstocking or shortages.
Fraud Detection: Banks and payment systems rely on fresh data to catch suspicious activity immediately.
In each case, fresh data helps businesses act quickly and stay proactive.
Vadym:
Got it! And that brings us to a question we hear a lot: what metrics should businesses use to measure data freshness? Are there key metrics to keep in mind?
Ievgen:
Yes, and this is really important. Here are seven key metrics businesses can use to evaluate data freshness:
Metric number 1 is Latency – how fast data moves from source to system.
The 2nd metric is Availability – making sure fresh data is ready when you need it.
The 3rd one is Completeness – no gaps in your data, so it’s reliable.
Metric number 4 is Consistency – data should be the same across systems.
Number 5 is Accuracy – because fresh data is only helpful if it’s correct.
Metric number 6 is Timeliness – are you getting the data at the right moments?
And the final metric number 7 is the Frequency of updates – how often data is refreshed.
These metrics help you gauge how fresh and reliable your data really is and is that aligned with your expectations…
Vadym:
That’s a great breakdown, Ievgen! Now, let’s talk about the challenges. What makes it difficult for businesses to keep their data fresh?
Ievgen:
There are a few big hurdles.
First, data silos—when data is scattered across platforms, it’s hard to consolidate quickly. Because you always need to make sure that all of the data for the specific report is already available,
If the joining would be done correctly,
If there is anything missing…
If you’re collecting the data from 5 or 7 sources just to consolidate in 1 report…
Let me just give you an example.
Website analytics data now is typically available in nearly real-time.
Whether business is using GA4 BigQuery Streaming Export or our accurate tracking with OWOX - it’s available very quickly.
But when it comes to the information from advertising platforms - that’s typically available just at midnight.
At 12 am of the specific ad account,
And different ad accounts might have different time zones…
And then some internal data, large enterprises can’t load all of the pieces together quickly, and there is a 1-day or so delay before the data about actual sales becomes available for being used in marketing reporting.
Vadym:
Ok, I see that data silos is one of those hurdles. So, what are the best practices to handle such situations?
Ievgen (continues):
So we at OWOX typically run all of the transformations related to web-analytics data to nail everything down at 7 am - close to the next working day.
Then there are resource limits—real-time data takes more infrastructure and sometimes staff… I haven’t seen the companies yet, who are growing their revenue as fast, as the amount of data they collect every single day.
This can slow things down if not managed well.
Vadym:
Right, so it’s all about balancing the speed you need with the resources you have. Ok, and what can businesses do to overcome these challenges?
Ievgen:
Here are some practical steps:
Step 1: Consolidate All the Data – Use a unified platform, like a data warehouse… Google BigQuery for example.
Step 2: Automate Data Collection. You don’t want to extract CSV files from your sources and then load them into a data warehouse. That leads to a lot of confusion and mistakes.
Plus Automation also reduces delays and keeps things flowing…
If you are watching us on YouTube, we’ll probably add a little tooltip here at the top and add a video on data collection in the description. It’s a step-by-step guide through the process with all of the possible ways to do this.
Vadym:
Thank you for sharing this guide with our audience.
Ok, so the first 2 steps are 1) Consolidate all the data and 2) Automate data collection… so, what is the next step after that?
Ievgen (continues):
Then we need to automate the data preparation as well. So Step #3 is about putting all of the data transformations on a schedule. Create those tables more suitable for reporting purposes than the raw data itself.
You don’t want every report to run through all of the data you have available.
Just once again, it’s expensive.
Step 4 is to Set the Reports Update Schedule.
Because it’s all linked together.
You collect the data on the specific time, you run those transformations, but then you need to deliver data to reports.
And you don’t need every report to be updated every hour.
As well as not every data point needs real-time updates.
So just check out which reports you need to be delivered weekly… maybe it’s like 4 pm every Thursday for a team meeting. But once again, you need to make sure that the data used in this report is already available by that time.
Which ones should be daily, let’s say at 7 am every day, or which specific reports should be updated in real-time or close to it, and you have no other choice than to minimize the number of data sources used there and reduce the time to data - time to decisions.
Vadym Kramarenko
Alright, we consolidated and automated our data, then we scheduled our transformations and then set the schedule for report updates.
What do we do next?
And last but not least. Step 5. It’s all about building a reliable and scalable data infrastructure.
Make sure your systems can handle growing data needs.
You will collect more and more data every day, just trust me.
And you need to be prepared.
Vadym:
That’s super helpful. And let’s touch quickly on costs—are there trade-offs to having fresher data?
Ievgen:
Absolutely, Vadym. Real-time data can be expensive in terms of tech and resources, so it’s important to ask, “Is the cost worth the benefit?”
Sometimes scheduled updates are enough, depending on your goals. You don’t have to go across the board in real time. You just need to fit the decision-making schedule. The data culture within each organization is different, so you need to fit.
Vadym:
Good point—it’s about picking your battles. So, where’s data freshness headed? Are there any trends shaping its future?
Ievgen:
For sure! We’re seeing more of the server-side tracking now. It typically comes with some kind of data streaming, so web-analytics data is not more or less available in real-time.
But then it’s another catch again,
As event-based GA4 data is not easily blendable with other sources…
But that’s probably a topic for one of our next episodes.
So yes, I see that trend of going server-side, most of our clients are heading there quickly.
Then, we’re seeing more predictive analytics—businesses using their data to not only react but anticipate.
And modern tools with or without AI is helping to access data faster too.
So these trends will make data freshness easier and more powerful.
Vadym:
That’s really exciting stuff! Thanks, Ievgen, for sharing so much useful information. Any final tips for our listeners?
Ievgen:
Just remember, data freshness is all about matching data speed to your business needs. Focus on quality as much as speed, and take it one step at a time.
Vadym:
Great advice. Thanks again, Ievgen! And for all our listeners, if you want to take your data insights to the next level, check out the OWOX BI marketing analytics suite linked below this episode.
Our platform can help you keep your data as fresh as YOU Need and ready for action.
You can schedule processes for automatic updates and get access to accurate data at any time of the day. Whether it's tracking market trends, monitoring customer behavior, or optimizing operations, OWOX BI provides the tools needed to keep data fresh and relevant.
You can start your free trial at owox.com… and if you have any questions reach out to our wonderful team to get help.
Don’t forget to subscribe to The Data Crunch Podcast for more insights!Ievgen:
Thanks, Vadym, and thanks to everyone listening. Keep exploring the possibilities with your data, and see you in one of the next episodes!
Make Sense of Your Data
Automatically generate Pivots & Charts in Google Sheets!
...plus, it's 100% Free!