Setting Up Advanced Analytics for a Mining Company: Hiveon Success Story
Daryna Kostrytsia, Lead Product Analyst at Hiveon
Yevheniia Hryshchenko, Project Manager at OWOX BI
Olga Mirgorodskaya, Creative writer @ OWOX
Get in-depth insights
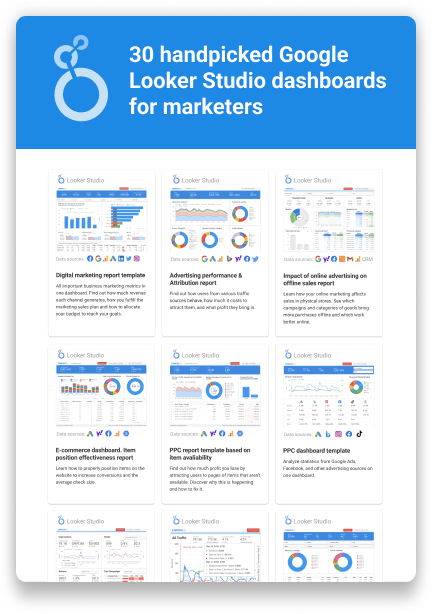
Get in-depth insights
Top 30 Handpicked Google Looker Studio Dashboards for Marketers
Hiveon, a mining ecosystem company, shares how they built an affordable and flexible tool for rapid marketing analysis with the help of OWOX BI and the OWOX Analytics team.
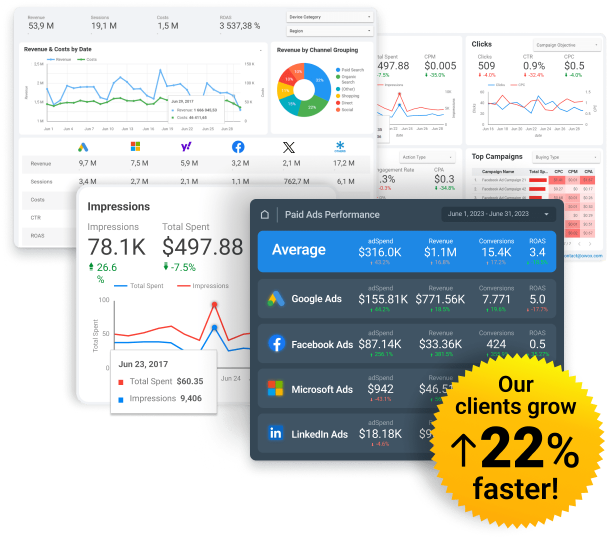
Gain clarity for better decisions without chaos
No switching between platforms. Get the reports you need to focus on campaign optimization
The Company
Founded in 2017, Hiveon offers the leading all-in-one cryptocurrency mining solution, with 2 million users worldwide. The Hiveon ecosystem currently includes Hiveon OS, Hiveon Pool, Hiveon ASIC Firmware, and Hiveon ASIC Hub. All Hiveon products are built around synergy, safety, and stability.
Hiveon OS is one of the first operating systems for cryptocurrency mining. It was created in accordance with the company’s goal to simplify technically complex solutions for users, making the blockchain accessible and understandable. This is what has helped Hiveon build a large, loyal community that helps the company improve its products with their feedback and initiative.
The Tasks
At Hiveon, we needed an affordable and flexible tool for:
- Quickly analyzing traffic sources. Yes, we do not have a lot of paid advertising, but it is not the main source of traffic for us, because it is not a source of traffic specific to our niche. It was important to analyze the funnel for organic, direct, and (especially) social media traffic. We have a large community, and a lot of activities take place, for example, on Twitter.
- Behavioral analytics — everything related to the website and in-product funnels. This is incredibly valuable knowledge that our business receives and uses to improve the UX and to identify and fix gaps in the product funnel.
In addition, we have enough retrospective data to search for insights and patterns, and we use this knowledge to plan the company’s future development. Google Analytics has limited data processing capabilities. Therefore, in order to free the hands of our analysts in terms of data access, we decided to use OWOX BI.
The Problems
Our product is complex and we currently have several domains across which it was difficult to set up cross-domain tracking. When users moved from one domain to another, the original client_id was lost.
Another problem was reaching the Google Analytics limit of 50,000 transactions per day. In the transaction report in Google Analytics, after the limit has been reached, the value '(other)' appears instead of the corresponding transaction_id.
The Solution
To create our Performance report, we decided to use OWOX BI and Google BigQuery.
Why we chose Google BigQuery for data collection and storage:
- Relative ease of setup for our use case
- Existing expertise of analysts in working with GBQ
- Possibility to integrate with visualization tools
How we combine data for reports in Google Big Query:
- With the help of OWOX BI Streaming, we collect raw, unsampled user behavior data from the website and transfer it to BigQuery;
- Transaction data from the website is sent to OWOX BI Streaming via the Measurement Protocol;
- Collected data is processed in Google BigQuery and displayed on dashboards in Google Looker Studio and Tableau using a built-in connector.
Colleagues from OWOX showed us how to set up cross-domain tracking. In addition to data collection, the OWOX team helped us with several interesting analytical tasks.
Defining new users based on first payment
In our case, in order to define a new user, we form an auxiliary table with transactions at the user level. That is, at the user level, we have a corresponding array of transactions. We can select from this auxiliary table sessions during which a first payment was made and set their corresponding status (0 or 1). After that, we connect the main table of streaming data with the auxiliary table of transactions by session id. Next, we can count the number of users who made a first payment using a formula. We count the number of unique users (client_id) if we have a session with the first payment.
Categorizing income based on service type
Since the project includes replenishing an online wallet, paying service fees, and withdrawing users’ income, it’s advisable to analyze the amounts of these transactions separately. For this, the Hiveon team added a product attribute to each transaction, and when preparing the report, the OWOX team was able to use this attribute both as a filter and for creating different metrics for each type of income.
The results
Thanks to the solution implemented, we managed to:
- Form a better understanding of how users use different domains and how they move among them;
- Increase the accuracy of determining a user’s first login to the site;
- Tie the user’s first payment to a specific traffic source;
- Determine the type of user based on the time of their first payment;
- More accurately determine conversions at different stages as well as on different website pages by receiving a unique OWOX User ID;
- Overcome the Google Analytics limit of 50,000 transactions per day, as transactions are recorded in full with OWOX BI Streaming
Thanks to OWOX BI, we now have an affordable and flexible tool for rapid analysis, which in turn allows us to quickly respond to changes.
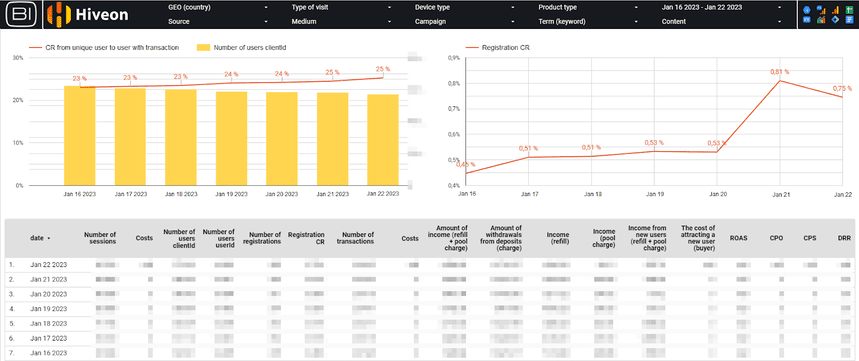
The main users of the report are data analysts who draw conclusions about the site’s operation and traffic performance. This report helps us understand the true composition of new users and returning users. Of course, Google Analytics also provides such information, but due to the more precise definition of the user, our report structure has changed. Conversion indicators, which are now determined by the new unique OWOX User ID, and the structure of traffic by source have also changed.
A great improvement from OWOX was the creation of intermediate tables and calculations that helped build the key metrics in the way we have chosen for our ecosystem. It should be noted that they are not standard, and therefore do not come out of the box.
Daryna Kostrytsia, Lead Product Analyst at Hiveon
However, as mentioned above, we went a little further and, with some modifications, transferred the report to Tableau (since it’s our main BI tool and it’s convenient to be able to have all our reports in one place). Thanks to OWOX BI, we were able to adapt the report to our own needs.
With OWOX BI, we managed to:
- Save time on collecting and processing data. Using reports that were built with the help of Google BigQuery, it became easier to monitor changes in web traffic, user activity, as well as certain types of marketing activities;
- Analyze user behavior in detail, which directly affected the reliability of results and the ability to search for insights. Certain elements of the site were analyzed for the first time, allowing us to approach the design of pages more thoughtfully and effectively.
Next Steps
In the future, we will be transitioning to Google Analytics 4, which means reconfiguring all tracking in Google Tag Manager because the data schemas in GA Universal and GA4 are significantly different. We expect to ask our colleagues from OWOX for help with this.
Get in-depth insights
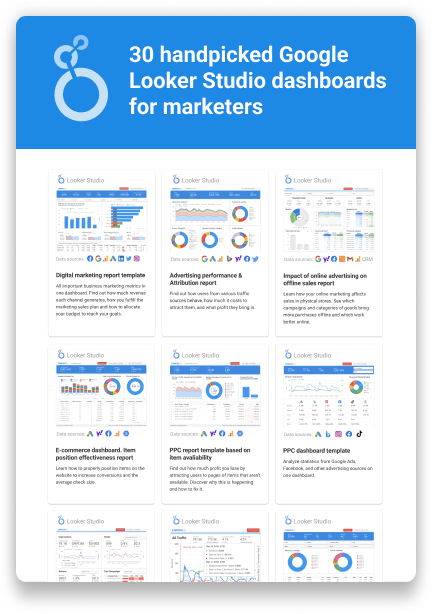
Get in-depth insights
Top 30 Handpicked Google Looker Studio Dashboards for Marketers